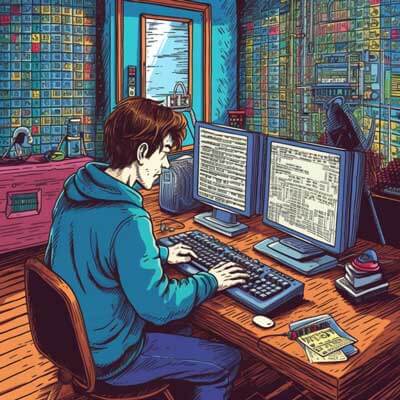
Table of Contents
Introduction
In Python, the Pandas library provides useful tools for data manipulation and analysis. One common task when working with data is to change the data type of a column. This can be useful when the current data type of a column is not appropriate for the analysis or when you want to optimize memory usage. In this answer, we will explore different methods to change the column type in Pandas.
Related Article: Creating Random Strings with Letters & Digits in Python
Method 1: Using the astype() method
The easiest way to change the data type of a column in Pandas is by using the astype() method. This method allows you to specify the new data type using a string representation. Here is an example:
import pandas as pd # Create a DataFrame data = {'Name': ['John', 'Jane', 'Mike'], 'Age': [25, 30, 35], 'Height': [1.75, 1.68, 1.82]} df = pd.DataFrame(data) # Display the original DataFrame print("Original DataFrame:") print(df) # Change the data type of the Age column to float df['Age'] = df['Age'].astype(float) # Display the modified DataFrame print("Modified DataFrame:") print(df)
Output:
Original DataFrame: Name Age Height 0 John 25 1.75 1 Jane 30 1.68 2 Mike 35 1.82 Modified DataFrame: Name Age Height 0 John 25.0 1.75 1 Jane 30.0 1.68 2 Mike 35.0 1.82
In the above example, we created a DataFrame with three columns: Name, Age, and Height. We then used the astype() method to change the data type of the Age column to float. Finally, we displayed the modified DataFrame.
Method 2: Using the to_numeric() function
Another way to change the data type of a column in Pandas is by using the to_numeric() function. This function allows you to convert a column to a numeric data type, such as integer or float. If the conversion fails for any value in the column, an error will be raised. Here is an example:
import pandas as pd # Create a DataFrame data = {'Name': ['John', 'Jane', 'Mike'], 'Age': ['25', '30', '35'], 'Height': ['1.75', '1.68', '1.82']} df = pd.DataFrame(data) # Display the original DataFrame print("Original DataFrame:") print(df) # Change the data type of the Age column to integer df['Age'] = pd.to_numeric(df['Age'], errors='coerce').astype(int) # Display the modified DataFrame print("Modified DataFrame:") print(df)
Output:
Original DataFrame: Name Age Height 0 John 25 1.75 1 Jane 30 1.68 2 Mike 35 1.82 Modified DataFrame: Name Age Height 0 John 25 1.75 1 Jane 30 1.68 2 Mike 35 1.82
In the above example, we created a DataFrame with three columns: Name, Age, and Height. We then used the to_numeric() function to convert the values in the Age column to integers. The errors='coerce' parameter allows any non-numeric value to be converted to NaN (Not a Number). Finally, we used the astype() method to change the data type of the Age column to integer.
Best Practices
When changing the column type in Pandas, it is important to consider the following best practices:
- Make sure to handle missing or non-numeric values appropriately. The errors parameter of the to_numeric() function can be set to 'coerce' to convert non-numeric values to NaN, or 'ignore' to leave them as they are.
- Be aware of potential data loss when converting between data types. For example, converting a float column to an integer column will truncate the decimal part of the values.
- Use the astype() method for simple data type conversions, such as changing an integer column to a float column. Use the to_numeric() function for more complex conversions that involve handling missing or non-numeric values.