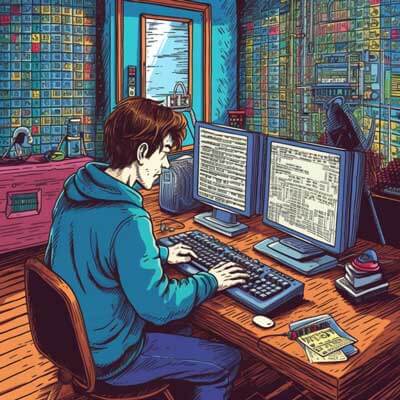
Table of Contents
To create and fill an empty Pandas DataFrame in Python, you can follow the steps outlined below.
Step 1: Importing the Required Libraries
The first step is to import the necessary libraries. In this case, you will need to import the Pandas library.
import pandas as pd
Related Article: How to Parallelize a Simple Python Loop
Step 2: Creating an Empty DataFrame
To create an empty DataFrame, you can use the pd.<a href="/how-to-select-multiple-columns-in-a-pandas-dataframe/">DataFrame()
function without passing any data or specifying column names. This will create an empty DataFrame with no rows or columns.
df = pd.DataFrame()
Step 3: Adding Columns to the DataFrame
Once you have created an empty DataFrame, you can add columns to it. There are several ways to add columns to a DataFrame, such as using a dictionary, a list, or a Series.
Adding Columns using a Dictionary:
You can add columns to a DataFrame by passing a dictionary to the pd.DataFrame()
function. The keys of the dictionary represent the column names, and the values represent the data for each column.
data = {'Name': ['John', 'Jane', 'Mike'], 'Age': [25, 30, 35], 'City': ['New York', 'London', 'Paris']} df = pd.DataFrame(data)
Adding Columns using a List:
Another way to add columns to a DataFrame is by using a list. Each element in the list represents the data for a column. You can then assign the list to a new column name.
names = ['John', 'Jane', 'Mike'] ages = [25, 30, 35] cities = ['New York', 'London', 'Paris'] df['Name'] = names df['Age'] = ages df['City'] = cities
Adding Columns using a Series:
You can also add columns to a DataFrame using a Pandas Series. A Series is a one-dimensional labeled array that can hold any data type.
names = pd.Series(['John', 'Jane', 'Mike']) ages = pd.Series([25, 30, 35]) cities = pd.Series(['New York', 'London', 'Paris']) df['Name'] = names df['Age'] = ages df['City'] = cities
Step 4: Filling the DataFrame with Rows
After creating an empty DataFrame and adding columns to it, you can fill the DataFrame with rows. There are multiple ways to achieve this, such as appending rows or creating a DataFrame from a list of dictionaries.
Appending Rows:
You can append rows to an existing DataFrame using the df.append()
method. This method takes another DataFrame or a dictionary as input and appends it to the original DataFrame.
new_data = {'Name': 'Sarah', 'Age': 28, 'City': 'Berlin'} df = df.append(new_data, ignore_index=True)
Creating a DataFrame from a List of Dictionaries:
Another way to fill a DataFrame with rows is by creating a new DataFrame from a list of dictionaries. Each dictionary in the list represents a row, where the keys correspond to the column names and the values represent the data for each column.
new_data = [{'Name': 'Sarah', 'Age': 28, 'City': 'Berlin'}, {'Name': 'Tom', 'Age': 32, 'City': 'Tokyo'}] df = pd.DataFrame(new_data)
Related Article: PHP vs Python: How to Choose the Right Language
Step 5: Best Practices and Alternative Ideas
- When creating an empty DataFrame, it is often useful to define the column names and data types beforehand. This can be done by passing the columns
parameter to the pd.DataFrame()
function with a list of column names.
df = pd.DataFrame(columns=['Name', 'Age', 'City'])
- If you have a large amount of data to add to a DataFrame, it may be more efficient to create a list of dictionaries first and then create the DataFrame in one go using the pd.DataFrame()
function. This can be faster than appending rows individually.
data = [{'Name': 'John', 'Age': 25, 'City': 'New York'}, {'Name': 'Jane', 'Age': 30, 'City': 'London'}, {'Name': 'Mike', 'Age': 35, 'City': 'Paris'}] df = pd.DataFrame(data)
- If you need to fill a DataFrame with random data, you can use the NumPy library to generate random values. For example, you can create an empty DataFrame with specific column names and then fill it with random numbers using the np.random.rand()
function.
import numpy as np df = pd.DataFrame(columns=['A', 'B', 'C']) df['A'] = np.random.rand(100) df['B'] = np.random.rand(100) df['C'] = np.random.rand(100)