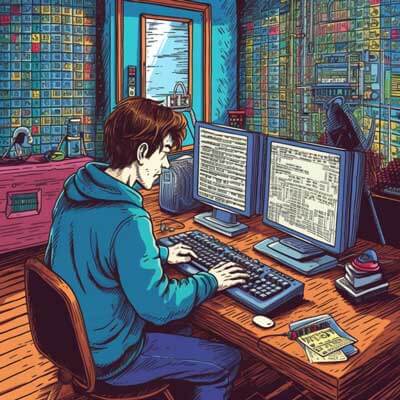
Table of Contents
In Python, the truth value of an array with more than one element is ambiguous. This means that when we try to evaluate the truthiness of an array, Python cannot determine a single boolean value for the entire array. Instead, we need to use the any()
or all()
functions to handle this ambiguity.
Why is the question asked?
The question of how to handle ambiguous truth values in Python arrays arises because Python treats arrays as objects and evaluates their truthiness based on their underlying data. When an array contains multiple elements, it becomes difficult to determine a single boolean value that represents the truthiness of the entire array.
For example, consider an array arr
that contains the values [0, 1, 2]
. If we were to evaluate the truthiness of arr
using a simple if statement like if arr:
, Python would not know whether to consider the array as True
or False
because it contains both non-zero and zero elements.
Related Article: How to Use the And/Or Operator in Python Regular Expressions
Potential reasons for ambiguous truth values
There are several potential reasons why an array in Python may have ambiguous truth values:
1. Mixed data types: If an array contains elements of different data types, it becomes challenging to determine a single boolean value that represents the entire array. For example, an array that contains both integers and strings would have an ambiguous truth value.
2. Numerical values: When an array contains numerical values, Python evaluates their truthiness based on their numeric values. This can lead to ambiguity when the array contains both zero and non-zero elements.
3. Logical operators: The use of logical operators (and
, or
, not
) with arrays can also result in ambiguous truth values. For example, if an array is used in a logical and
operation and it contains both True
and False
elements, the resulting truth value may not be clear.
Using the any()
and all()
functions
To handle ambiguous truth values in Python arrays, we can use the any()
and all()
functions. These functions provide a way to evaluate the truthiness of an array based on the values of its elements.
- The any()
function returns True
if at least one element in the array evaluates to True
. Otherwise, it returns False
.
- The all()
function returns True
if all elements in the array evaluate to True
. Otherwise, it returns False
.
By using these functions, we can explicitly handle the ambiguity and make informed decisions based on the desired logic.
Example 1: Using any()
Suppose we have an array arr
that contains the values [0, 1, 2]
. If we want to check if at least one element in the array is non-zero, we can use the any()
function as follows:
arr = [0, 1, 2] if any(arr): print("At least one element is non-zero") else: print("All elements are zero")
In this example, the any()
function checks if any element in the array is non-zero. Since the array contains the value 1
, which is non-zero, the condition evaluates to True
, and the corresponding message is printed.
Example 2: Using all()
Suppose we have another array arr
that contains the values [1, 1, 1]
. If we want to check if all elements in the array are non-zero, we can use the all()
function as follows:
arr = [1, 1, 1] if all(arr): print("All elements are non-zero") else: print("At least one element is zero")
In this example, the all()
function checks if all elements in the array are non-zero. Since all elements in the array are non-zero, the condition evaluates to True
, and the corresponding message is printed.
Suggestions and alternative ideas
When dealing with ambiguous truth values in Python arrays, here are some suggestions and alternative ideas:
1. Explicitly handle the ambiguity: Instead of relying on the default truthiness evaluation of Python, use the any()
or all()
functions to explicitly handle the ambiguity and make your intentions clear in the code.
2. Use numpy arrays: If you are working with numerical data and need to perform complex operations on arrays, consider using the numpy
library. Numpy arrays provide enhanced functionality for handling numerical data, including handling ambiguous truth values.
3. Convert arrays to boolean: If you only need to check if an array contains any non-zero elements, you can convert the array to a boolean value using the bool()
function. This will evaluate the truthiness of the array based on whether it contains any non-zero elements.
4. Consider alternative data structures: If the ambiguity of truth values in arrays becomes a significant issue in your code, consider using alternative data structures that do not have this ambiguity. For example, you could use sets or dictionaries to represent collections of values instead of arrays.
Related Article: Python Sort Dictionary Tutorial
Best practices
When handling ambiguous truth values in Python arrays, it is important to follow these best practices:
1. Be explicit: Use the any()
or all()
functions to explicitly handle the ambiguity and make your intentions clear in the code. This helps improve code readability and reduces the chance of unexpected behavior.
2. Document your intentions: If your code relies on ambiguous truth values for arrays, make sure to document this behavior in comments or documentation. This helps other developers understand the logic behind your code and avoid confusion.
3. Test edge cases: When working with arrays, test your code with different scenarios, including arrays with different data types and combinations of zero and non-zero elements. This ensures that your code behaves as expected in various situations.