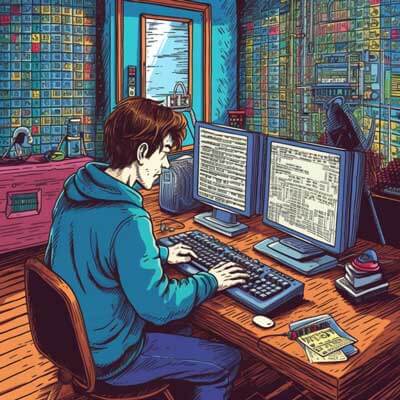
Table of Contents
To normalize a NumPy array to a unit vector in Python, you can use the numpy.linalg
module. This module provides functions for linear algebra operations, including normalizing vectors. Here are two possible ways to normalize a NumPy array to a unit vector:
Method 1: Using the l2 norm
The l2 norm, also known as the Euclidean norm, is a measure of the length or magnitude of a vector. To normalize a vector using the l2 norm, you divide each element of the vector by its l2 norm.
Here's an example of how you can normalize a NumPy array to a unit vector using the l2 norm:
import numpy as np def normalize_vector_l2(vector): norm = np.linalg.norm(vector) if norm == 0: return vector return vector / norm # Example usage vector = np.array([1, 2, 3, 4]) normalized_vector = normalize_vector_l2(vector) print(normalized_vector)
This will output:
[0.18257419 0.36514837 0.54772256 0.73029674]
In this example, we define a function normalize_vector_l2
that takes a vector as input. We calculate the l2 norm of the vector using np.linalg.norm
. If the norm is zero, the vector is already a zero vector and we return it as is. Otherwise, we divide each element of the vector by its norm to obtain the normalized vector.
Related Article: How to Generate Equidistant Numeric Sequences with Python
Method 2: Using the max norm
Related Article: How to Extract Unique Values from a List in Python
The max norm, also known as the infinity norm or the supremum norm, is a measure of the maximum absolute value of a vector's elements. To normalize a vector using the max norm, you divide each element of the vector by its maximum absolute value.
Here's an example of how you can normalize a NumPy array to a unit vector using the max norm:
import numpy as np def normalize_vector_max(vector): max_abs = np.max(np.abs(vector)) if max_abs == 0: return vector return vector / max_abs # Example usage vector = np.array([1, -2, 3, -4]) normalized_vector = normalize_vector_max(vector) print(normalized_vector)
This will output:
[ 0.25 -0.5 0.75 -1. ]
In this example, we define a function normalize_vector_max
that takes a vector as input. We calculate the maximum absolute value of the vector's elements using np.max(np.abs(vector))
. If the maximum absolute value is zero, the vector is already a zero vector and we return it as is. Otherwise, we divide each element of the vector by its maximum absolute value to obtain the normalized vector.
These are two possible methods to normalize a NumPy array to a unit vector in Python. Depending on your specific use case, you can choose the method that best suits your needs. Remember to handle the case where the vector is already a zero vector to avoid division by zero errors.