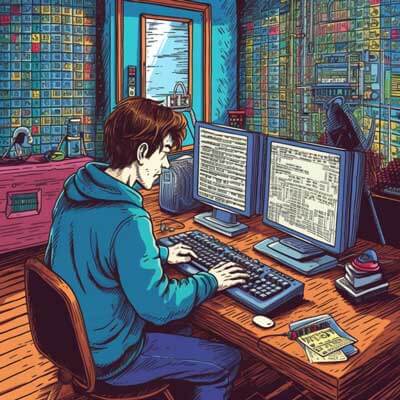
Table of Contents
Parallelizing a loop in Python can greatly improve the performance of your code, especially when dealing with computationally intensive tasks or large datasets. In this guide, we will explore different approaches to parallelizing a simple Python loop and discuss some best practices. Let's get started!
1. Using the concurrent.futures module
One way to parallelize a loop in Python is by using the
concurrent.futures
module, which provides a high-level interface for asynchronously executing callables. This module introduces the ThreadPoolExecutor
and ProcessPoolExecutor
classes, which allow us to execute tasks concurrently using threads or processes, respectively.
To parallelize a loop using
ThreadPoolExecutor
, you can follow these steps:
1. Import the necessary modules:
import concurrent.futures
2. Create a
ThreadPoolExecutor
object:
with concurrent.futures.ThreadPoolExecutor() as executor:
3. Define a function that represents the task to be executed in parallel. This function should take an input parameter that represents the loop variable:
def task(i): # Do some computation here return result
4. Submit the tasks to the executor using the
submit()
method, passing the task function and the loop variable as arguments:
future = executor.submit(task, i)
5. Collect the results using the
result()
method, which blocks until the task is complete and returns the result:
result = future.result()
Here's an example that demonstrates the parallelization of a simple loop using
ThreadPoolExecutor
:
import concurrent.futures def task(i): # Do some computation here return i * 2 with concurrent.futures.ThreadPoolExecutor() as executor: # Submit tasks to the executor futures = [executor.submit(task, i) for i in range(10)] # Collect the results results = [future.result() for future in concurrent.futures.as_completed(futures)] print(results)
This example creates a
ThreadPoolExecutor
, submits 10 tasks to the executor, and collects the results as they become available. Note that the order of the results may vary, as they are processed concurrently.
Related Article: How to Use Slicing in Python And Extract a Portion of a List
2. Using the multiprocessing module
Another way to parallelize a loop in Python is by using the
multiprocessing
module, which allows you to spawn multiple processes to perform tasks in parallel. This approach is particularly useful for CPU-bound tasks, as it takes advantage of multiple CPU cores.
To parallelize a loop using
multiprocessing
, you can follow these steps:
1. Import the necessary modules:
import multiprocessing
2. Define a function that represents the task to be executed in parallel. This function should take an input parameter that represents the loop variable:
def task(i): # Do some computation here return result
3. Create a
Pool
object:
pool = multiprocessing.Pool()
4. Map the task function to a range of values using the
map()
method. This will distribute the tasks across multiple processes:
results = pool.map(task, range(10))
Here's an example that demonstrates the parallelization of a simple loop using
multiprocessing
:
import multiprocessing def task(i): # Do some computation here return i * 2 if __name__ == '__main__': with multiprocessing.Pool() as pool: results = pool.map(task, range(10)) print(results)
This example creates a
Pool
, maps the task
function to a range of values, and collects the results. The if __name__ == '__main__':
guard is used to prevent infinite recursion when running the script as a module.
Best practices and considerations
When parallelizing a loop in Python, there are a few best practices and considerations to keep in mind:
- Ensure that the tasks you are parallelizing are truly independent and do not have any shared state. Parallelizing tasks with shared state can lead to data races and incorrect results.
- Be aware of the Global Interpreter Lock (GIL) in CPython, which prevents multiple native threads from executing Python bytecodes in parallel. This means that parallelizing CPU-bound tasks using threads may not result in significant performance improvements in CPython. However, parallelizing I/O-bound tasks can still provide performance benefits.
- Test the performance of your parallelized code using different numbers of threads or processes to find the optimal configuration for your specific use case. Too many threads or processes can lead to increased overhead and decreased performance due to context switching.
- Consider using libraries such as NumPy, pandas, or Dask, which provide built-in support for parallel operations on arrays and dataframes.
- Take advantage of any available optimizations provided by the libraries you are using. For example, NumPy provides vectorized operations that can significantly improve performance compared to explicit loops.
- Monitor the resource usage of your parallelized code, especially when using a large number of threads or processes. Excessive resource usage can lead to decreased performance or even system instability.
Alternative approaches
In addition to the
concurrent.futures
and multiprocessing
modules, there are other libraries and frameworks available for parallelizing Python code, such as:
-
joblib
: A library that provides high-level parallel computing capabilities, with support for both local and distributed computing.
-
Ray
: A general-purpose framework for parallel and distributed Python applications, with support for task parallelism, distributed computing, and distributed data processing.
-
Dask
: A flexible library for parallel computing in Python, with support for parallelizing operations on large datasets and distributed computing.
These libraries offer additional features and functionalities that may be useful depending on your specific use case. Be sure to explore their documentation and examples to determine which one best suits your needs.