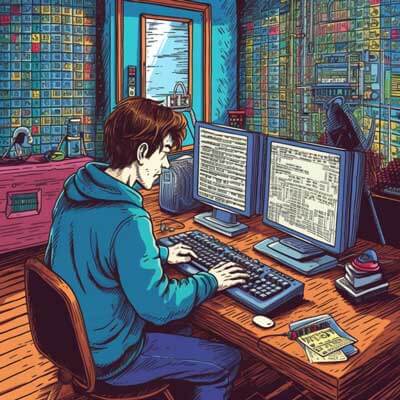
Table of Contents
Pandas is a useful data manipulation library in Python that provides various functionalities for data analysis. One of its key features is the ability to perform groupby operations, which allows you to group data based on one or more columns and compute statistics for each group. In this article, we will explore how to use the groupby function in Pandas to perform group statistics in Python.
Step 1: Import the necessary libraries
First, you need to import the necessary libraries. In this case, you will need to import the pandas library:
import pandas as pd
Related Article: How to Generate Equidistant Numeric Sequences with Python
Step 2: Load the data
Next, you need to load the data into a Pandas DataFrame. You can do this by reading a CSV file, an Excel file, or any other supported file format. For the purpose of this example, let's assume you have a CSV file named "data.csv" that contains the following data:
Name,Gender,Age,Salary John,Male,25,50000 Jane,Female,30,60000 Mark,Male,35,70000 Emily,Female,40,80000
You can load this data into a DataFrame using the read_csv
function:
data = pd.read_csv('data.csv')
Step 3: Group the data
Once you have loaded the data, you can use the groupby
function to group the data based on one or more columns. The groupby
function returns a GroupBy
object, which allows you to perform various aggregate operations on each group.
For example, if you want to group the data by gender, you can do the following:
grouped_data = data.groupby('Gender')
This will group the data into two groups: one for males and one for females.
Step 4: Compute statistics for each group
Once you have grouped the data, you can compute statistics for each group. The GroupBy
object provides several methods for computing statistics, such as mean
, sum
, min
, max
, and count
.
For example, if you want to compute the mean age for each gender group, you can use the mean
method:
mean_age = grouped_data['Age'].mean()
This will compute the mean age for each gender group and return a Series object with the results.
Similarly, you can compute other statistics by using the appropriate method. For example, to compute the total salary for each gender group, you can use the sum
method:
total_salary = grouped_data['Salary'].sum()
This will compute the total salary for each gender group and return a Series object with the results.
Related Article: How to Use 'In' in a Python If Statement
Step 5: Display the results
Finally, you can display the results by printing the computed statistics. You can use the print
function to do this:
print(mean_age) print(total_salary)
This will print the mean age and total salary for each gender group.
Alternative: Aggregating multiple columns
In addition to computing statistics for a single column, you can also aggregate multiple columns at once. To do this, you can pass a list of column names to the groupby
function.
For example, if you want to compute the mean age and total salary for each gender group, you can do the following:
grouped_data = data.groupby('Gender')['Age', 'Salary'] mean_age_salary = grouped_data.mean()
This will compute the mean age and total salary for each gender group and return a DataFrame object with the results.
Best practices
When using the groupby
function in Pandas, it is important to keep the following best practices in mind:
1. Make sure the columns you want to group by are categorical or discrete variables. Grouping by continuous variables may not yield meaningful results.
2. Consider sorting the data before performing the groupby operation. This can help in cases where you want to compute statistics that depend on the order of the data, such as cumulative sums.
3. Use the reset_index
method to convert the grouped data into a DataFrame if you want to perform further operations on the grouped data.
4. Take advantage of the various methods available on the GroupBy
object, such as apply
and transform
, to perform custom aggregations or transformations.